95% Confidently Incorrect
How to interpret one of the most widely misunderstood results in medicine
Yes, this post is about statistics - BUT WAIT! Give it a chance - I promise there are no formulas or maths, and you might find it useful, or better yet, interesting!
This is all about the 95% confidence interval (95% CI). For those who are unfamiliar, this is a statistic which provides a range of uncertainty around the main result (referred to as a point estimate) of a study. For example, a study might show that medication X reduces your chance of dying after a heart attack with a relative risk (RR) point estimate of 0.8, and a 95% CI of 0.7 to 0.9.
The 95% CI is one of the most misunderstood results in statistics, and one of the main reasons for this is it is usually taught wrong.
The common understanding of a 95% confidence interval is that there is a 95% chance the true result lies within that range. Let me begin by saying this is false.
However, most explanations of why it is false are unintuitive, and sometimes seem like fastidious and irrelevant technicalities. That is a shame, because in my opinion there is an intuitive way of explaining why this is false, and why it is important to understand it is false.
Why doesn’t a 95% CI mean this?
It’s much easier to explain what a 95% CI doesn’t mean than what it does, so we will start with that. Central to understanding this result is understanding 2 differing philosophies of probability, both of which you probably understand intuitively, but may not have considered as being separate.
Frequentist philosophy is based on understanding of the number of times an outcome occurs under multiple repetitions. For example, rolling a dice or tossing a coin multiple times, or in biomedicine, random sampling from a population or repeating an experiment. It says, “when I repeat this thing over and over again, I expect X proportion of the results to be Y (or more/less than Y)”.
Bayesian philosophy is based on a subjective probability of an outcome occurring, usually in a specific instance which may not be repeatable. For example, the probability it will rain today or that England will win the World cup this year. Importantly, Bayesian probability is an expression of belief, and incorporates prior beliefs (referred to simple as “priors”) into this subjective probability which are updated as new data is gathered. It says, “Adding in this data to what I knew before, I believe there is a X% chance that true result is Y (or more/less than Y)”.
A 95% CI is explicitly a frequentist expression of probability. This means, that under multiple repetitions of a particular experiment, 95% of ALL the confidence intervals would contain the true result. This is NOT the same as making a subjective (Bayesian) probability statement about any single 95% CI that it has a 95% chance of containing the true result.
To show how these two similar sounding things can be very different, here is an extreme example.
Let’s take a completely discredited therapy - Vitamin C has failed to show any evidence of efficacy for reducing mortality from sepsis in any randomised trials. We more-or-less know that it is not meaningfully effective and the true effect size is ~0.
Let’s say I somehow still manage to get ethical approval to do another trial of Vitamin C for sepsis. By random chance, the result of my study is that the patients treated with Vitamin C had a lower rate of mortality, with RR 0.8, 95% CI 0.7 - 0.9. Is there a 95% chance that the true effect lies between 0.7 and 0.9? No! Of course not!
We already know that this treatment doesn’t reduce mortality. There is a much lower subjective probability of the true result being within this range, in fact it is closer to 0% than 95%! This is despite the fact that it remains true that if we repeated this experiment over and over again, we would expect 95% of all the CIs to contain the true effect.
The key point here is that when making a subjective statement about probability, we have to take into account what is already known. We are not simply making a statement about what we expect under repetition (which is what a 95% CI means), we are making a statement about what we believe to be true in that specific instance. If we very strongly believe the result, we might have a stronger subjective probability than 95%. Equally, we may have reason to strongly doubt the result (as in the example) in which case our subjective probability will be much lower.
At the risk of adding some confusion, it is possible that the frequentist and subjective probability statements can align. This would occur in a scenario where you know absolutely nothing about the experiment, and so can incorporate no prior information into your subjective probability. In this case, all you know is that the long run probability of the 95% CIs is that 95% of them will contain the true result, and in the case when this is the only information available to inform a subjective probability statement, your subjective probability of the 95% CI containing the true result will be 95% (for those interested, this is the intuitive explanation for why when using a Bayesian analysis with an uninformative prior, the 95% credible interval will be exactly the same as the frequentist 95% confidence interval).
In reality, the likelihood you would be interpreting a specific 95% CI in the context of absolutely no information about the experiment at all is so small as to be irrelevant, and this is why it is important we dispel the notion that the probability that a single given 95% CI automatically has a 95% probability of containing the true result. This subjective probability statement is heavily influenced by what we already know, or believe to be true.
What DOES a 95% CI mean?
This is a very difficult question and is even a point of much discussion amongst statisticians. I will not claim some infallible truth to the meaning of a 95% CI, but will try to explain some important philosophical and technical points on its interpretation.
It is true that a 95% CI has the long run characteristic of 95% of them containing the true result under repetition - but this phenomenon is almost a distraction, because as we’ve seen, it is almost irrelevant in interpreting any particular 95% CI. The purpose of a 95% CI is simply to give a range of potential true effects which would be compatible with your observed data.
The technical way to understand this range, is to imagine the hypothesis you are testing is: The true effect is equal the point estimate you observed. The P value for the point estimate would then be 1 (that is, under repetition, you would expect 100% of the observed results to be as, or more extreme than the point estimate - because it is the true effect). The 95% CI then spreads out to either side until it reaches the results which would have a P value of 0.05 (that is, if the true effect is your point estimate, then which result (or more extreme) would you expect to observe 5% of the time or less). These results either side of the point estimate give you your 95% CI.
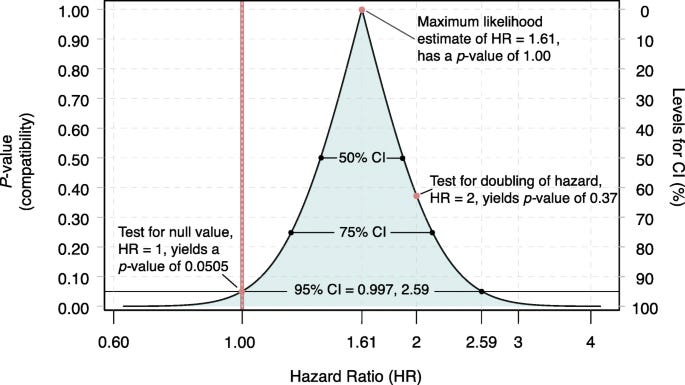
This technical explanation may sound confusing, and is not intuitive. To expand more on this point you can read some excellent papers:
Rafi et al 2020: Semantic and cognitive tools to aid statistical science: replace confidence and significance by compatibility and surprise
Amrhein et al 2022: Discuss practical importance of results based on interval estimates and p-value functions, not only on point estimates and null p-values
It is perhaps not so important to get hung up on the precise technical aspects of a 95% CI, but to consider it more generally as an indication of uncertainty. It is simply the range of effects which are most compatible with the data you have observed.
For further reading, an excellent “Head to head” discussion from The BMJ can be read here:
Gelman and Greenland 2019: Are confidence intervals better termed “uncertainty intervals”?
Conclusion
If some of this has made your head hurt, don’t worry. The specific nuts and bolts are not too important as long as you can take away the following important points
In the long run under multiple repetitions, 95% of 95% CIs will contain the true effect
The subjective probability of a particular 95% CI containing the true result is not automatically 95%, as it is influenced by what you already knew to be true before the experiment
A 95% CI is providing a range of results which are most compatible with the data you observed in the experiment, and is an important indicator of uncertainty around the point estimate
Thank you, very enlightening. So do we need to also pay attention to how wide this 95%CI is or just to whether it crosses the 1 as we usually do?
Really interesting. Are there theories or explanations for the basis in the gap between the CI and the subjective probability? Is it tied to the experimental design?